How artificial intelligence is transforming enterprise applications
Artificial intelligence (AI) is no longer a futuristic concept; it’s rapidly reshaping the enterprise landscape. From streamlining workflows to revolutionizing data analysis and personalizing customer experiences, AI is fundamentally altering how businesses operate. This transformation isn’t just about incremental improvements; it’s about unlocking unprecedented levels of efficiency, gaining deeper insights, and creating more engaging customer interactions. This exploration delves into the multifaceted ways AI is impacting enterprise applications across various sectors.
The integration of AI is driving significant changes in how businesses manage their operations, interact with customers, and analyze data. We’ll examine the practical applications of AI in various enterprise software, highlighting the benefits and challenges associated with this technological shift. This includes exploring the ethical considerations and potential pitfalls to ensure responsible AI implementation within organizations.
Enhanced Efficiency and Productivity in Enterprise Applications
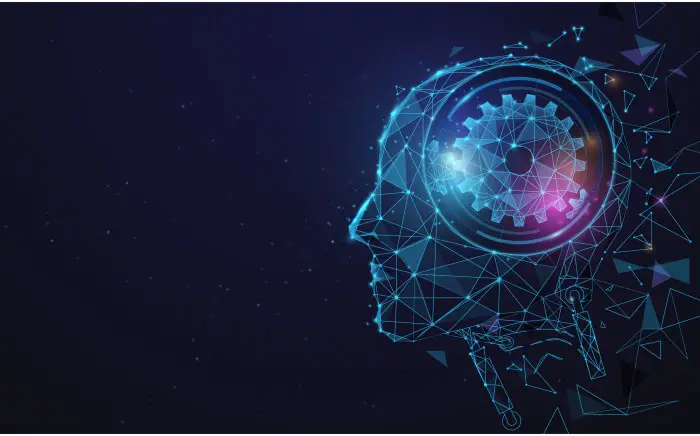
Source: ashlingpartners.com
Artificial intelligence (AI) is revolutionizing enterprise applications, significantly boosting efficiency and productivity across various sectors. AI-powered automation, predictive analytics, and intelligent chatbots are transforming workflows, improving decision-making, and enhancing customer experiences. This leads to streamlined operations, reduced costs, and improved overall business performance.
AI-Powered Automation Streamlining Workflows
AI is automating repetitive and time-consuming tasks across numerous enterprise applications, freeing up human employees to focus on more strategic initiatives. This automation results in increased efficiency and improved accuracy. The following table illustrates the impact of AI-driven automation on various application types:
Application Type | Before AI | After AI | Percentage Improvement |
---|---|---|---|
CRM (Customer Relationship Management) | Manual data entry, lead qualification, and customer segmentation; slow response times to customer inquiries. | Automated lead scoring, personalized marketing campaigns, and automated customer service responses; faster response times and improved customer engagement. | 30-50% (depending on implementation and complexity) |
ERP (Enterprise Resource Planning) | Manual reconciliation of financial data, slow inventory management, and inefficient procurement processes. | Automated financial reporting, real-time inventory tracking, and automated procurement; reduced errors and improved resource allocation. | 25-40% (depending on implementation and complexity) |
Supply Chain Management | Manual order processing, forecasting based on historical data, and reactive responses to supply chain disruptions. | Automated order fulfillment, predictive forecasting using machine learning, and proactive risk management; optimized inventory levels and reduced delays. | 15-30% (depending on implementation and complexity) |
Human Resources | Manual recruitment processes, time-consuming onboarding procedures, and inefficient performance management. | Automated candidate screening, AI-powered onboarding systems, and automated performance reviews; faster hiring processes and improved employee engagement. | 20-35% (depending on implementation and complexity) |
AI-Driven Predictive Analytics in Supply Chain Management
AI-driven predictive analytics significantly enhances decision-making within supply chain management applications. By analyzing vast amounts of data, AI algorithms can identify patterns and predict future trends, allowing businesses to proactively address potential issues and optimize their operations. Examples include:
- Predicting demand fluctuations: AI can analyze historical sales data, market trends, and external factors (e.g., weather patterns, economic indicators) to accurately forecast future demand, enabling businesses to optimize inventory levels and avoid stockouts or overstocking.
- Optimizing logistics and transportation: AI can analyze real-time data on traffic, weather, and other factors to optimize delivery routes, reduce transportation costs, and improve delivery times.
- Identifying potential supply chain disruptions: AI can monitor various data sources (e.g., news feeds, social media, weather reports) to identify potential risks, such as natural disasters or geopolitical events, allowing businesses to proactively mitigate disruptions.
- Improving inventory management: AI can analyze sales data, lead times, and other factors to optimize inventory levels, reducing storage costs and minimizing the risk of stockouts.
AI-Powered Chatbots Enhancing Customer Service
AI-powered chatbots are transforming customer service and support within enterprise applications, providing 24/7 availability and instant responses to customer inquiries. This leads to improved response times, increased customer satisfaction, and reduced operational costs. For example, a leading e-commerce company reported a 30% reduction in customer service call volume after implementing an AI-powered chatbot, while simultaneously experiencing a 15% increase in customer satisfaction scores. The chatbot handled simple inquiries, freeing up human agents to focus on more complex issues. Furthermore, the ability to provide immediate responses, regardless of time zone or day of the week, significantly enhances customer experience and loyalty.
Revolutionizing Data Analysis and Insights within Enterprises
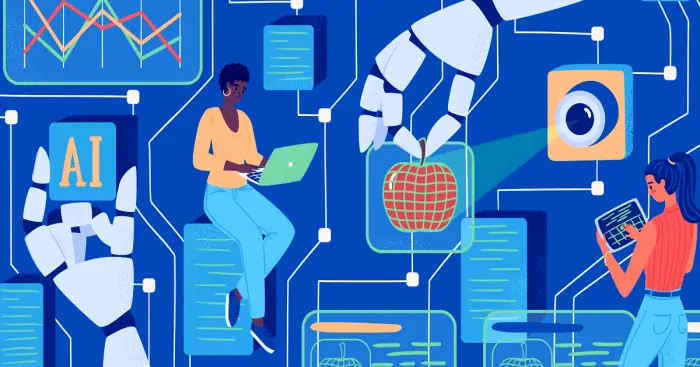
Source: builtin.com
The integration of artificial intelligence (AI) is fundamentally reshaping how enterprises handle and interpret data. Traditional methods, while valuable, often struggle with the sheer volume, velocity, and variety of data generated in today’s digital landscape. AI-powered techniques offer a transformative solution, enabling businesses to extract deeper insights, make faster decisions, and gain a significant competitive advantage. This section explores the revolution AI is driving in enterprise data analysis and the resulting improvements in insight generation.
Traditional vs. AI-Powered Data Analysis Methods
The shift from traditional data analysis to AI-powered approaches represents a significant leap in capability. Traditional methods often rely on manual processes and limited analytical tools, while AI leverages sophisticated algorithms and machine learning to unlock hidden patterns and predictions. The following table highlights key differences:
Traditional Methods | AI-Powered Methods |
---|---|
Relies heavily on manual data cleaning and preparation. | Automates data cleaning, transformation, and feature engineering. |
Limited ability to handle large datasets. | Scales effectively to handle big data volumes and complexities. |
Focuses on descriptive statistics and pre-defined analyses. | Enables predictive and prescriptive analytics through machine learning. |
Insights are often delayed due to manual processing. | Provides real-time or near real-time insights. |
Prone to human error and bias in analysis. | Reduces human bias and improves accuracy through algorithmic objectivity. |
Machine Learning in Fraud Detection and Risk Management
Machine learning algorithms play a crucial role in identifying subtle patterns and anomalies within enterprise data, significantly enhancing fraud detection and risk management capabilities. These algorithms can analyze vast datasets to uncover hidden relationships and predict future events with greater accuracy than traditional methods.
The importance of machine learning in this context cannot be overstated. By identifying anomalies and predicting potential risks, organizations can proactively mitigate threats and protect their assets.
- Fraud Detection in Financial Transactions: Machine learning models can analyze transaction data to identify unusual patterns indicative of fraudulent activity, such as unusually large transactions or transactions originating from unusual locations. For example, a model might flag a credit card transaction from a country the cardholder has never visited before.
- Credit Risk Assessment: AI algorithms can assess the creditworthiness of loan applicants by analyzing various factors such as credit history, income, and employment status, leading to more accurate risk assessments and improved lending decisions. This can help reduce the likelihood of loan defaults.
- Insurance Claim Fraud Detection: Machine learning can identify patterns in insurance claims that suggest fraudulent activity, such as inconsistencies in the reported details or unusually high claim amounts for similar incidents. This leads to faster detection and reduced payouts for fraudulent claims.
AI-Enhanced Data Visualization and Reporting in Financial Reporting Software
Imagine a financial reporting software enhanced with AI. Instead of static tables and charts, the software dynamically generates interactive dashboards that provide a comprehensive overview of key financial metrics. These dashboards could incorporate:
* Interactive Geographic Maps: Visualizing sales performance across different regions, highlighting high-performing and underperforming areas with color-coded regions and interactive drill-down capabilities to explore detailed data for each region.
* Predictive Trend Lines: AI algorithms could project future revenue, expenses, and profits based on historical data and current market trends. These projections would be displayed as dynamic trend lines on the dashboard, providing valuable insights into future financial performance.
* Automated Report Generation: The software could automatically generate customized reports based on user-defined parameters and preferences. These reports would be delivered in an easily digestible format, such as interactive dashboards or concise summaries, eliminating the need for manual report creation.
* Anomaly Detection Highlights: The system would automatically highlight any significant deviations from expected trends or patterns, alerting users to potential problems or opportunities. For example, an unexpected spike in expenses in a particular department could be flagged immediately, allowing for prompt investigation.
This AI-powered financial reporting software would provide a significantly improved user experience, leading to faster decision-making and better financial management. The interactive nature of the visualizations would allow users to explore data in greater depth, uncovering hidden insights and gaining a more comprehensive understanding of the financial health of the organization.
Transforming Customer Experiences and Personalization
Artificial intelligence is revolutionizing the way businesses interact with their customers, moving beyond generic marketing strategies to highly personalized experiences that foster loyalty and drive revenue. This shift is particularly evident in e-commerce and marketing automation, where AI algorithms analyze vast amounts of data to understand individual customer preferences and behaviors, enabling businesses to deliver tailored content, offers, and services.
AI-driven personalization significantly enhances customer engagement and satisfaction by creating a more relevant and valuable experience. This leads to increased customer lifetime value and a stronger competitive advantage in today’s dynamic marketplace. The following sections detail how AI achieves this transformation.
Personalized Customer Experiences in E-commerce and Marketing Automation
AI facilitates personalized experiences across various touchpoints within the customer journey. In e-commerce, AI-powered platforms analyze browsing history, purchase behavior, and demographic data to offer personalized product recommendations, targeted advertising, and customized website layouts. Marketing automation tools leverage AI to segment audiences based on their characteristics and interactions, allowing for the delivery of tailored email campaigns, social media content, and other marketing messages.
Personalization Strategy | AI-Driven Implementation | Example |
---|---|---|
Personalized Product Recommendations | Collaborative filtering, content-based filtering, hybrid recommendation systems analyzing user data, purchase history, and product attributes. | Amazon recommending products based on past purchases and browsing history. |
Targeted Advertising | Machine learning models predicting customer preferences and likelihood of purchase, enabling targeted ad placement across various channels. | Google Ads using AI to optimize ad targeting and bidding based on user demographics and behavior. |
Customized Website Experiences | AI-powered personalization engines adapting website content, layout, and product displays based on individual user profiles. | Netflix dynamically adjusting its interface and recommendations based on user viewing history. |
Personalized Email Marketing | AI-driven segmentation and content personalization, optimizing email subject lines, content, and call-to-actions based on user preferences. | Marketing automation platforms like HubSpot using AI to personalize email campaigns based on user behavior and demographics. |
AI-Powered Recommendation Engines and Enhanced Customer Engagement
AI-powered recommendation engines are a cornerstone of personalized customer experiences. These systems analyze vast datasets to predict which products or services a customer is most likely to be interested in, significantly improving customer engagement and driving sales.
The effectiveness of these engines hinges on the ability to accurately predict customer preferences and deliver relevant recommendations. Different types of recommendation systems exist, each with its strengths and weaknesses.
- Content-Based Filtering: Recommends items similar to those a user has previously interacted with. For example, if a user likes a particular genre of music, the system will recommend other artists or albums within that genre.
- Collaborative Filtering: Recommends items based on the preferences of users with similar tastes. For example, if users A and B both liked the same movies, the system might recommend movies liked by user A to user B, and vice versa.
- Hybrid Recommendation Systems: Combine content-based and collaborative filtering techniques to leverage the strengths of both approaches. This often leads to more accurate and diverse recommendations.
- Knowledge-Based Systems: Use explicit knowledge about items and user preferences to generate recommendations. For example, a travel recommendation system might use knowledge about a user’s desired destination, budget, and travel style to suggest suitable hotels and tours.
Ethical Considerations in AI-Driven Personalization
The use of AI in personalizing customer experiences raises several ethical considerations that require careful attention. Transparency and responsible data handling are paramount.
- Data Privacy: Collecting and using customer data for personalization requires adherence to strict privacy regulations, such as GDPR and CCPA. Customers must be informed about how their data is being collected and used, and given control over their data.
- Bias Mitigation: AI algorithms can inherit and amplify biases present in the data they are trained on, leading to unfair or discriminatory outcomes. Efforts must be made to identify and mitigate these biases to ensure fairness and equity.
- Transparency and Explainability: Customers should understand how AI is being used to personalize their experiences. Explainable AI (XAI) techniques can help make the decision-making process of AI algorithms more transparent and understandable.
- Data Security: Robust security measures are essential to protect customer data from unauthorized access and breaches.