How Machine Learning Improves Enterprise-Level Customer Insights
Unlocking the true potential of your customer data is no longer a futuristic fantasy; it’s a reality powered by machine learning. Enterprise businesses are increasingly leveraging the power of AI to move beyond simple data analysis and into the realm of predictive insights. This allows for a deeper understanding of customer behavior, preferences, and lifetime value, ultimately driving more effective marketing strategies, personalized experiences, and data-driven business decisions. This exploration delves into the transformative impact of machine learning on enterprise-level customer understanding.
From sophisticated customer segmentation and precise targeting based on behavioral patterns to proactive customer service and optimized pricing strategies, machine learning offers a comprehensive suite of tools for businesses aiming to elevate their customer relationships and boost profitability. We’ll examine real-world examples, explore various machine learning models, and uncover the key performance indicators (KPIs) that are significantly enhanced through the application of this transformative technology.
Enhanced Customer Segmentation & Targeting
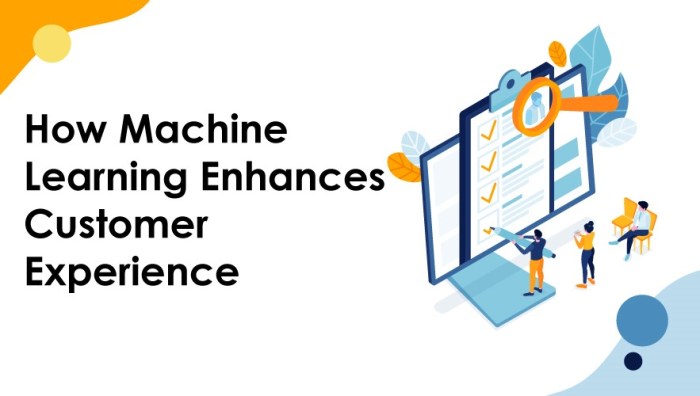
Source: smallbizdaily.com
Machine learning significantly enhances customer segmentation and targeting by enabling businesses to move beyond basic demographic data and delve into the complexities of customer behavior. By analyzing vast datasets encompassing purchasing history, website interactions, social media activity, and more, machine learning algorithms identify subtle patterns and relationships that traditional methods often miss. This leads to more precise customer groupings and highly effective, personalized marketing strategies.
Machine learning algorithms, specifically unsupervised learning techniques like k-means clustering and hierarchical clustering, are instrumental in grouping customers based on shared characteristics. These algorithms analyze diverse behavioral and demographic data points to identify natural clusters or segments within the customer base. This allows for a deeper understanding of customer needs and preferences, paving the way for targeted marketing campaigns that resonate with each segment.
Customer Segmentation Based on Machine Learning
The following table illustrates how machine learning can segment customers based on various data points, leading to tailored marketing approaches and improved response rates. These segments are hypothetical examples and could vary significantly based on the specific data and business context.
Customer Segment | Defining Characteristics | Marketing Strategies | Predicted Response Rate |
---|---|---|---|
High-Value Loyalists | High purchase frequency, high average order value, long tenure, positive reviews | Exclusive offers, personalized recommendations, loyalty programs, early access to new products | 70-80% |
Price-Sensitive Shoppers | Low average order value, infrequent purchases, sensitivity to discounts and promotions | Targeted discounts, promotional emails, flash sales, competitive pricing strategies | 40-50% |
Potential High-Value Customers | Recent high-value purchases, frequent website visits, engagement with premium product pages | Upselling opportunities, personalized product recommendations, targeted email sequences | 50-60% |
At-Risk Customers | Decreased purchase frequency, low engagement, negative reviews or feedback | Win-back campaigns, personalized offers, improved customer service interactions | 20-30% |
Predicting Customer Lifetime Value (CLTV)
Machine learning models are highly effective in predicting customer lifetime value (CLTV), a crucial metric for resource allocation and strategic decision-making. These models consider factors such as purchase history, demographics, engagement levels, and churn risk to estimate the total revenue a customer is expected to generate throughout their relationship with the business. For example, a model might predict that a specific customer segment, based on their online behavior and purchase patterns, has a high CLTV, warranting increased investment in personalized marketing and retention strategies.
Consider a subscription-based software company. By using machine learning to predict CLTV, they can identify high-value customers who are likely to renew their subscriptions. This allows them to prioritize customer support and proactive engagement with these customers, potentially reducing churn and maximizing revenue. Conversely, customers with low predicted CLTV might receive less intensive support, allowing the company to optimize resource allocation towards high-potential clients.
Marketing Campaign Targeting a Specific Segment
Let’s consider a marketing campaign targeted at the “Potential High-Value Customers” segment identified above. This segment exhibits promising characteristics, indicating a high likelihood of future substantial purchases.
Campaign Objectives: Increase conversion rates among potential high-value customers, drive upselling and cross-selling opportunities, and enhance customer lifetime value.
Channels: Personalized email marketing, targeted social media advertising, retargeting ads based on website browsing history.
Anticipated Results: A 20% increase in conversion rates, a 15% increase in average order value, and a 10% improvement in customer retention within the targeted segment. This success would be measured through A/B testing comparing the campaign’s performance against a control group.
Improved Customer Experience & Personalization
Machine learning (ML) significantly enhances customer experience by enabling businesses to deliver personalized interactions at scale, moving beyond generic offerings to create truly individualised experiences that foster loyalty and drive revenue. This personalization extends across various touchpoints, from product recommendations to proactive customer service interventions.
ML algorithms analyze vast amounts of customer data – browsing history, purchase patterns, demographics, and interactions – to build detailed customer profiles. This granular understanding allows for the creation of highly targeted and relevant experiences, leading to increased customer satisfaction and engagement.
Personalized Recommendations and Content Delivery
The ability to deliver personalized recommendations and content is a cornerstone of improved customer experience. By analyzing past behavior and preferences, ML models predict what customers are most likely to want next, leading to increased sales and engagement.
- E-commerce: A clothing retailer uses ML to recommend products based on a customer’s browsing history, past purchases, and similar customers’ buying patterns. This results in a more relevant shopping experience, increasing conversion rates.
- Streaming Services: Netflix and Spotify leverage ML to suggest movies, TV shows, and music based on individual viewing and listening habits. This personalization keeps users engaged and reduces churn.
- Financial Services: Banks utilize ML to recommend financial products, such as investments or loans, tailored to a customer’s financial profile and risk tolerance.
Natural Language Processing for Customer Feedback Analysis
Natural Language Processing (NLP) plays a crucial role in understanding and acting upon customer feedback. By analyzing unstructured data like surveys, reviews, and social media comments, NLP algorithms can extract valuable insights into customer sentiment, pain points, and unmet needs. This information is invaluable for product development, service improvement, and proactive issue resolution.
For example, a sentiment analysis tool powered by NLP can identify negative trends in customer reviews about a particular product feature. This allows the company to address the issue promptly, preventing further negative feedback and potential customer churn. Topic modeling can also group similar feedback themes together, providing a concise summary of prevalent customer concerns. This data-driven approach ensures that improvements are focused on areas that truly matter to customers.
Proactive Customer Service Issue Resolution
ML algorithms can anticipate and address customer service issues before they escalate into negative experiences. By analyzing historical data on support tickets, customer interactions, and product usage patterns, ML models can identify potential problems and trigger proactive interventions.
Imagine a scenario where an ML model detects a significant increase in error reports related to a specific software feature. The system can automatically alert the support team, allowing them to proactively investigate the issue, deploy a patch, and communicate with affected customers before they experience significant disruption. This proactive approach minimizes customer frustration and enhances their overall experience. Furthermore, predictive models can identify customers at high risk of churning based on their recent behavior and interactions, allowing the company to offer targeted retention strategies.
Predictive Analytics for Business Decisions

Source: entireweb.com
Machine learning empowers enterprises to move beyond descriptive analytics, offering predictive capabilities that significantly impact strategic decision-making. By leveraging historical customer data and applying sophisticated algorithms, businesses can anticipate future trends and behaviors, enabling proactive interventions and optimized resource allocation. This section will explore how different machine learning models contribute to predictive analytics, focusing specifically on customer churn prediction and the optimization of pricing strategies.
Predictive Modeling for Customer Churn
Predictive modeling plays a crucial role in identifying customers at high risk of churn. Several machine learning models excel in this area, each with its strengths and weaknesses. The following table compares the predictive capabilities of regression and classification models in forecasting customer churn.
Model Type | Accuracy | Limitations |
---|---|---|
Logistic Regression | Provides probability scores indicating churn likelihood. Relatively simple to implement and interpret. Accuracy depends heavily on data quality and feature engineering. | Assumes a linear relationship between features and the outcome. May not capture complex interactions within the data. |
Support Vector Machines (SVM) | Effective in high-dimensional spaces and can handle non-linear relationships. Can achieve high accuracy with proper parameter tuning. | Computationally expensive for large datasets. Model interpretation can be challenging. Requires careful selection of kernel functions. |
Random Forest | Robust to outliers and handles high dimensionality well. Provides feature importance scores for better understanding of drivers of churn. | Can be computationally intensive for very large datasets. Model interpretation can be less straightforward compared to logistic regression. |
Gradient Boosting Machines (GBM) | Often achieves high accuracy. Can capture complex interactions between features. | Prone to overfitting if not properly regularized. Requires careful hyperparameter tuning. |
Key Performance Indicators (KPIs) Improved by Machine Learning
Applying machine learning to customer data directly improves several critical KPIs, providing valuable insights into business performance and guiding strategic adjustments.
Below are some examples:
- Customer Churn Rate: By accurately predicting which customers are likely to churn, businesses can proactively implement retention strategies, such as targeted discounts or personalized communication, leading to a lower churn rate and increased customer lifetime value. For example, a telecommunications company using a machine learning model might identify 10% of their subscribers as high-risk for churn. By implementing a targeted retention campaign, they might reduce this percentage to 5%, resulting in significant revenue savings.
- Customer Acquisition Cost (CAC): Machine learning can optimize marketing campaigns by identifying the most promising customer segments, leading to more efficient targeting and a lower CAC. For instance, a retail company could use machine learning to identify potential customers with a high propensity to purchase their products, resulting in a more effective and cost-efficient marketing campaign.
- Customer Lifetime Value (CLTV): By better understanding customer behavior and predicting future purchases, businesses can increase CLTV. A subscription service, for example, might use machine learning to predict which customers are likely to upgrade to a premium plan, allowing them to target those customers with tailored upselling campaigns.
- Net Promoter Score (NPS): Predictive models can identify factors driving customer satisfaction and dissatisfaction, enabling businesses to proactively address issues and improve the overall customer experience, thus increasing NPS. A software company might use machine learning to analyze customer support interactions and identify common pain points, allowing them to improve their product or service and ultimately enhance customer satisfaction.
Optimizing Pricing Strategies with Machine Learning
Machine learning enables businesses to dynamically adjust pricing based on real-time customer behavior and market trends. This process typically involves several steps:
- Data Collection and Preparation: Gathering relevant data, including historical sales data, customer demographics, competitor pricing, and market trends. This data is then cleaned, preprocessed, and formatted for use in machine learning models.
- Model Development and Training: Developing and training a machine learning model, such as a regression model, to predict optimal pricing based on the prepared data. This model considers various factors influencing price elasticity, such as customer segment, product features, and competitive landscape.
- Price Optimization and Implementation: Using the trained model to generate optimized pricing recommendations. This might involve setting different prices for different customer segments or adjusting prices in response to real-time market fluctuations.
- Monitoring and Evaluation: Continuously monitoring the performance of the pricing strategy and retraining the model periodically to incorporate new data and adapt to changing market conditions. Key metrics to track include revenue, profit margins, and customer retention.
For example, an e-commerce company could use machine learning to dynamically adjust the price of a particular product based on demand, competitor pricing, and customer segment. During peak demand periods, the price might increase slightly, while during periods of low demand, the price might be reduced to stimulate sales. This dynamic pricing strategy could lead to increased revenue and profit margins while maintaining customer satisfaction.