Top-rated SaaS solutions for enterprise-level data analytics
Unlocking the power of data is paramount for modern enterprises. The right data analytics SaaS solution can transform raw information into actionable insights, driving strategic decision-making and fueling growth. But with a plethora of options available, choosing the ideal platform can feel overwhelming. This comprehensive guide navigates the complexities of enterprise-level data analytics, comparing top-rated SaaS solutions to help you make an informed choice.
We’ll delve into key features, pricing models, and target users, examining strengths and weaknesses related to data integration, scalability, and security. Critical considerations for selection, including data volume, integration needs, user experience, and budget, will be addressed. Finally, real-world case studies will illustrate successful enterprise deployments, offering valuable lessons and best practices for implementation.
Top Tier Data Analytics Platforms
Enterprise-level data analytics requires robust and scalable solutions capable of handling massive datasets and providing actionable insights. Choosing the right platform is crucial for success, and this overview compares five leading contenders, examining their strengths and weaknesses across key features. The selection considers factors vital for enterprise adoption, including data integration, scalability, security, and pricing models.
Top Tier Data Analytics Platforms: A Comparative Overview
The following table compares five leading enterprise-level data analytics SaaS solutions across key features, pricing models, and target users. Note that pricing can vary significantly based on data volume, user numbers, and specific features selected.
Solution Name | Key Features | Pricing Model | Target User |
---|---|---|---|
Snowflake | Cloud-based data warehousing, scalable compute, data sharing capabilities, advanced analytics, strong security features. | Pay-as-you-go, based on compute and storage consumption. | Large enterprises, data scientists, analysts requiring high scalability and performance. |
Databricks | Unified analytics platform combining data warehousing, data lakehouse, and machine learning capabilities, collaborative workspace. | Subscription-based, tiered pricing based on cluster size and usage. | Data engineers, data scientists, analysts working with large datasets and needing collaborative environments. |
Google BigQuery | Serverless, highly scalable data warehouse, SQL-based querying, machine learning integration, geospatial analysis. | Pay-as-you-go, based on query processing and storage. | Organizations with large datasets needing fast query performance and serverless scalability. |
Amazon Redshift | Managed cloud data warehouse service, scalable performance, integration with other AWS services, robust security features. | Pay-as-you-go, based on compute and storage usage. | Enterprises already heavily invested in the AWS ecosystem needing a scalable data warehouse solution. |
Microsoft Azure Synapse Analytics | Unified analytics platform integrating data warehousing, data lake, and big data processing capabilities, strong integration with other Azure services. | Pay-as-you-go, tiered pricing based on compute and storage. | Organizations using Microsoft Azure services needing a comprehensive analytics platform. |
Data Integration Capabilities: Strengths and Weaknesses
Each platform offers diverse data integration capabilities, but their strengths and weaknesses vary. Snowflake excels with its ability to ingest data from various sources, including cloud storage, databases, and streaming platforms, using its Snowpipe feature. Databricks’ strength lies in its unified approach, allowing seamless integration between different data sources and processing engines. Google BigQuery provides robust connectors to many popular data sources, simplifying data ingestion. Amazon Redshift integrates seamlessly with other AWS services, making it a natural choice for AWS-centric environments. Azure Synapse Analytics offers similar integration strengths within the Microsoft Azure ecosystem. Weaknesses can arise from complexities in handling less common data formats or specific proprietary systems requiring custom connectors.
Scalability and Security Features
Scalability and security are paramount for enterprise-level data analytics. All five platforms offer highly scalable architectures capable of handling massive data volumes. Snowflake, BigQuery, and Redshift are particularly known for their serverless architectures, automatically scaling resources based on demand. Databricks and Azure Synapse Analytics provide options for both serverless and cluster-based scaling, offering flexibility based on specific needs. Security features include encryption at rest and in transit, access control mechanisms, and compliance certifications (e.g., SOC 2, ISO 27001). The specific certifications and security features offered vary slightly between platforms, so a thorough evaluation is crucial based on individual enterprise security requirements. For example, a financial institution might prioritize platforms with specific compliance certifications relevant to their industry regulations.
Key Considerations for Enterprise Data Analytics Solution Selection
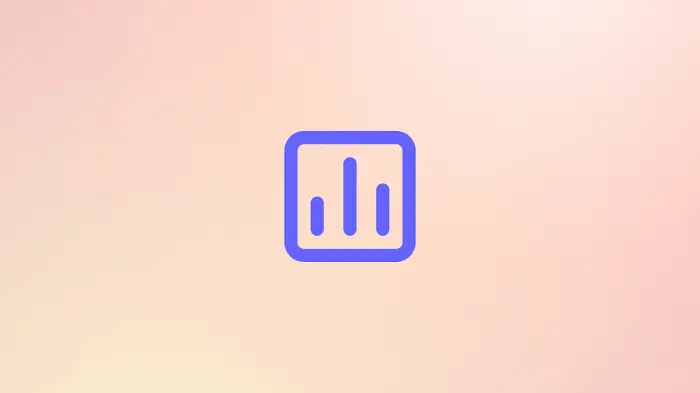
Source: medium.com
Selecting the right enterprise-level data analytics SaaS solution is a critical decision impacting operational efficiency, strategic decision-making, and overall business success. A poorly chosen solution can lead to wasted resources, inaccurate insights, and missed opportunities. Therefore, a thorough evaluation process is paramount, considering various factors beyond initial cost and features.
The selection process must be data-driven and strategic, aligning with the organization’s specific needs and long-term goals. This involves a comprehensive assessment of existing infrastructure, data volume and velocity, integration requirements, and the overall business objectives that the analytics solution is intended to support.
Critical Factors for Enterprise Data Analytics Solution Selection
Enterprises should carefully consider several key factors when evaluating potential SaaS solutions. A balanced approach, weighing technical capabilities against business needs and budgetary constraints, is essential for optimal selection.
- Data Volume and Velocity: The chosen solution must be capable of handling the current and projected volume and velocity of data. Consider factors like data ingestion rates, storage capacity, and processing power. For instance, a company experiencing rapid data growth should prioritize solutions with scalable architecture and robust data handling capabilities. Underestimating data volume can lead to performance bottlenecks and system failures.
- Integration Needs: Seamless integration with existing enterprise systems (CRM, ERP, marketing automation platforms, etc.) is crucial. The solution should offer robust APIs and connectors to ensure data flows smoothly between different systems. A lack of proper integration can result in data silos and hinder the generation of comprehensive insights.
- User Experience (UX): The platform’s usability and accessibility are paramount. A user-friendly interface ensures that business users of varying technical expertise can effectively utilize the platform and extract valuable insights. Poor UX can lead to low adoption rates and hinder the return on investment.
- Budget and Total Cost of Ownership (TCO): While initial licensing costs are important, enterprises must also consider ongoing maintenance, support, training, and potential consulting fees. A detailed TCO analysis helps in making informed budgetary decisions and avoiding unexpected expenses.
- Scalability and Flexibility: The solution should be able to adapt to the changing needs of the business. This includes the ability to scale up or down resources as needed, accommodate new data sources, and integrate new analytical tools or techniques.
Data Governance and Compliance in Solution Selection
Data governance and compliance are paramount in selecting an enterprise data analytics SaaS solution. The chosen solution must adhere to relevant data privacy regulations (GDPR, CCPA, etc.) and industry best practices. This includes ensuring data security, access control, and auditability.
Organizations must verify that the vendor’s security measures are robust and meet their specific compliance requirements. Features like data encryption, access controls, and data masking are crucial for protecting sensitive information. Furthermore, the vendor should provide transparent documentation regarding their security practices and compliance certifications.
Decision-Making Framework for SaaS Solution Evaluation
A structured decision-making framework helps enterprises systematically evaluate different SaaS solutions. This framework should incorporate a weighted scoring system that assigns priorities to the critical factors identified earlier.
Criterion | Weighting | Vendor A Score | Vendor B Score | Vendor C Score |
---|---|---|---|---|
Data Volume Handling | 30% | 8 | 7 | 9 |
Integration Capabilities | 25% | 7 | 9 | 6 |
User Experience | 20% | 9 | 8 | 7 |
Total Cost of Ownership | 15% | 6 | 7 | 8 |
Compliance and Security | 10% | 9 | 8 | 9 |
This example demonstrates a weighted scoring system. Each vendor is assessed based on the identified criteria, and the scores are weighted according to their relative importance. The vendor with the highest weighted average score is typically considered the most suitable option. This framework allows for a transparent and objective comparison of different solutions, facilitating a data-driven decision.
Case Studies

Source: userpilot.com
Successful enterprise-level deployments of data analytics SaaS solutions demonstrate the transformative power of these platforms. Analyzing these case studies reveals best practices for implementation and integration, offering valuable insights for organizations considering similar initiatives. The following examples highlight diverse applications and outcomes, showcasing the versatility of data analytics in addressing complex business challenges.
Case Study 1: Retail Giant Optimizes Supply Chain with Snowflake
This global retailer faced significant challenges in managing its vast and complex supply chain. Inaccurate forecasting led to stockouts and overstocking, resulting in substantial losses. They chose Snowflake, a cloud-based data warehouse, to consolidate data from various sources, including point-of-sale systems, inventory management systems, and third-party logistics providers.
The implementation involved migrating existing data into Snowflake, building a data pipeline for real-time data ingestion, and developing custom dashboards for visualizing key performance indicators (KPIs). The process was phased, starting with a pilot project focusing on a specific product category. This allowed the team to refine their processes and address any unforeseen issues before scaling the solution across the entire enterprise. Results included a 15% reduction in inventory holding costs, a 10% improvement in forecast accuracy, and a significant reduction in stockouts.
Case Study 2: Financial Institution Enhances Fraud Detection with Alteryx
A major financial institution struggled with escalating fraud rates, particularly in online transactions. Their existing fraud detection system was slow, inefficient, and lacked the analytical capabilities to identify sophisticated fraud schemes. They selected Alteryx, a self-service data analytics platform, to build a more robust and scalable fraud detection system.
The implementation focused on integrating Alteryx with the institution’s existing data sources, including transaction data, customer information, and geolocation data. Data scientists used Alteryx’s visual workflow tools to build predictive models that identified suspicious transactions with greater accuracy. The system was integrated into the bank’s existing security infrastructure, allowing for real-time fraud detection and prevention. This resulted in a 20% reduction in fraudulent transactions and a significant improvement in customer trust.
Case Study 3: Healthcare Provider Improves Patient Outcomes with Tableau
A large healthcare provider sought to improve patient outcomes and operational efficiency by leveraging data analytics. Their existing data was siloed across various departments, making it difficult to gain a comprehensive view of patient care. They adopted Tableau, a business intelligence platform, to consolidate and analyze patient data.
The implementation involved connecting Tableau to various data sources, including electronic health records (EHRs), billing systems, and patient surveys. Clinicians and administrators used Tableau’s intuitive interface to create dashboards and reports that provided insights into patient demographics, treatment outcomes, and operational performance. The resulting data-driven insights led to improvements in patient care coordination, reduced hospital readmissions, and increased operational efficiency.
Successful Data Analytics Implementation Workflow
A visual representation of a successful data analytics implementation in a large enterprise would depict a cyclical process. First, data ingestion would be shown, representing the collection of data from various sources, including databases, APIs, and cloud storage. This data would then flow into a data processing stage, where data cleansing, transformation, and enrichment occur. This processed data would then feed into the data analysis stage, where statistical modeling, machine learning, and other analytical techniques are applied to extract meaningful insights. Finally, these insights would be visualized through interactive dashboards and reports, enabling decision-makers to understand key trends and patterns. The cycle would then repeat, with insights informing future data collection and analysis, creating a continuous feedback loop for iterative improvement.